淡水生物和海水生物从基因到种群水平指标对毒性物质的敏感性差异研究——以铜为例
Study on the Sensitivity Differences of Indicators to Toxic Substances from Gene to Population Level in Freshwater and Marine Organisms: A Case Study of Copper
-
摘要: 物种敏感度分布(SSD)是一种广泛用于生态风险评价和水质基准建立的统计分布模型,目前用于SSD分析的主要是生物个体水平的毒性数据。随着基因、生化、细胞、器官以及种群水平毒性数据的日渐丰富,这些不同层次毒理指标数据能否应用于水质基准制定和生态风险评价值得研究。本研究搜集了铜对淡水和海水生物基因、生化、细胞、器官、个体和种群水平的毒性数据,构建了相应的SSD曲线,采用双样本K-S检验和5%物种受影响的浓度(HC5)差异法比较了不同指标间的差异,采用拐点分析法确定了构建稳定SSD所需的最小样本量。K-S检验结果表明,水生生物个体急性指标对铜的敏感性要显著低于其他指标,淡水生物的个体慢性指标的敏感性显著高于生化和种群指标。HC5差异法结果显示,海水生物不同指标对铜的敏感性顺序为:基因≥器官≥种群>个体慢性≥生化≥细胞>个体急性,淡水生物为:个体慢性 > 种群≥器官 > 生化≥基因≥细胞 > 个体急性。淡水和海水生物不同指标毒性数据构建稳定SSD所需最小样本量范围分别为5~22和5~17。由于毒性数据的质量和数量问题,水生生物不同层次指标对铜的敏感性并没有呈现出逐级响应的关系,基于现有数据尚难以大范围地支持其在水质基准制定和风险评价中的应用。本研究结果可为我国水质基准的后期修订和风险评价提供借鉴。Abstract: Toxicity data on individual levels were mainly used in the construction of species sensitivity distribution (SSD), which was a widely used statistical model for ecological risk assessment and water quality criteria establishment. With the toxicity data on levels of genes, biochemistry, cells, organs, and populations increasing, it is worthwhile to study whether these toxicological indicators are appropriate to be applied in the construction of water quality criteria and ecological risk assessment. In the present study, toxicity data of copper on the genetic, biochemical, cell, organ, individual and population levels in freshwater and marine organisms were collected, and the corresponding SSD curves were constructed. Differences between different endpoints were compared by the two-sample K-S test and the hazardous concentration for 5% of species (HC5) difference method. The change point analysis method was adopted to obtain the minimum sample size required for the construction of stable SSD. The results of K-S test showed that in aquatic organisms, the sensitivity of acute indicators was significantly lower than the others; in freshwater organisms, the sensitivity of individual chronic indicators was significantly higher than those of biochemical and population indicators. The order of sensitivity of different indicators to copper was: gene ≥ organ ≥ population > individual chronic ≥ biochemistry ≥ cell > individual acute indicators for marine organisms; and individual chronic > population ≥ organ > biochemistry ≥ gene ≥ cell > individual acute indicators for freshwater organisms. The minimum sample size required to construct a stable SSD for different toxicological endpoints toxicity data were 5~22 and 5~17 for freshwater and marine organisms, respectively. Due to the quality and quantity of toxicity data, we did not observe a hierarchical cascade of biological responses to stress for copper. Therefore, based on existing data, it is difficult to conclude that different toxicological endpoints can be applied in ecological risk assessment and establishment of water quality criteria on a large scale. Results of this study provide a reference for the revision of China’s water quality criteria and risk assessment.
-
United States Environmental Protection Agency. Guidelines for Deriving Numerical National Water Quality Criteria for the Protection of Aquatic Organisms and Their Uses[R]. Washington DC:Office of Research and Development, 1985:1-57 European Commission (EC). Technical Guidance Document on Risk Assessment[R]. Luxembourg:Office for Official Publications of the European Communities, 2003:149-150 Australian and New Zealand Environmentent and Conservation Council, and Agriculture and Resource Management Council of Australia and New Zealand (ANZECC & ARMCANZ). Australian and New Zealand Guidelines for Fresh and Marine Water Quality[R]. Canberra:ANZECC & ARMCANZ, 2000 Del Signore A, Hendriks A J, Lenders H J R, et al. Development and application of the SSD approach in scientific case studies for ecological risk assessment[J]. Environmental Toxicology and Chemistry, 2016, 35(9):2149-2161 中华人民共和国国家环境保护部. HJ 831-2017淡水水生生物水质基准制定技术指南[S]. 北京:中国环境科学出版社, 2017 Ministry of Environmental Protection of the People's Republic of China. HJ 831-2017 Technical Guideline for Deriving Water Quality Criteria for the Protection of Freshwater Aquatic Organisms[S]. Beijing:China Environmental Science Press, 2017 (in Chinese)
Fedorenkova A, Vonk J A, Lenders H J R, et al. Ecotoxicogenomics:Bridging the gap between genes and populations[J]. Environmental Science & Technology, 2010, 44(11):4328-4333 Caldwell D J, Mastrocco F, Hutchinson T H, et al. Derivation of an aquatic predicted no-effect concentration for the synthetic hormone, 17α-ethinyl estradiol[J]. Environmental Science & Technology, 2008, 42(19):7046-7054 Jin X W, Wang Y Y, Jin W, et al. Ecological risk of nonylphenol in China surface waters based on reproductive fitness[J]. Environmental Science & Technology, 2013, 48(2):1256-1262 高祥云, 李霁, 王晓南, 等. 基于生长发育毒性终点的国内外部分水体中全氟辛烷磺酸盐生态风险评价[J]. 环境化学, 2018, 37(8):1789-1795 Gao X Y, Li J, Wang X N, et al. Growth and development toxicity based ecological risk assessment of PFOS in freshwater of domestic and abroad[J]. Environmental Chemistry, 2018, 37(8):1789-1795(in Chinese)
Huang Q S, Bu Q W, Zhong W G, et al. Derivation of aquatic predicted no-effect concentration (PNEC) for ibuprofen and sulfamethoxazole based on various toxicity endpoints and the associated risks[J]. Chemosphere, 2018, 193:223-229 P é rez-Iglesias J M, Franco-Belussi L, Natale G S, et al. Biomarkers at different levels of organisation after atrazine formulation (SIPTRAN 500SC®) exposure in Rhinella schineideri (Anura:Bufonidae) Neotropical tadpoles[J]. Environmental Pollution, 2019, 244:733-746 Wo K T, Lam P K S, Wu R S S. A comparison of growth biomarkers for assessing sublethal effects of cadmium on a marine gastropod, Nassarius festivus[J]. Marine Pollution Bulletin, 1999, 39(1-12):165-173 Spurgeon D J, Ricketts H, Svendsen C, et al. Hierarchical responses of soil invertebrates (earthworms) to toxic metal stress[J]. Environmental Science & Technology, 2005, 39(14):5327-5334 刘娜, 金小伟, 王业耀, 等. 生态毒理数据筛查与评价准则研究[J]. 生态毒理学报, 2016, 11(3):1-10 Liu N, Jin X W, Wang Y Y, et al. Review of criteria for screening and evaluating ecotoxicity data[J]. Asian Journal of Ecotoxicology, 2016, 11(3):1-10(in Chinese)
Yan Z G, Yang N Y, Wang X N, et al. Preliminary analysis of species sensitivity distribution based on gene expression effect[J]. Science China:Earth Sciences, 2012, 55(6):907-913 Nichols J W, Breen M, Denver R J, et al. Predicting chemical impacts on vertebrate endocrine systems[J]. Environmental Toxicology and Chemistry, 2011, 30(1):39-51 Lin B L, Tokai A, Nakanishi J. Approaches for establishing predicted-no-effect concentrations for population-level ecological risk assessment in the context of chemical substances management[J]. Environmental Science & Technology, 2005, 39(13):4833-4840 Iwasaki Y, Hayashi T I, Kamo M. Estimating population-level HC5 for copper using a species sensitivity distribution approach[J]. Environmental Toxicology and Chemistry, 2013, 32(6):1396-1402 金小伟, 王子健, 王业耀, 等. 淡水水生态基准方法学研究:繁殖/生殖毒性类化合物水生态基准探讨[J]. 生态毒理学报, 2015, 10(1):31-39 Jin X W, Wang Z J, Wang Y Y, et al. Methodologies for deriving aquatic life criteria (ALC):Discussion of ALC for chemicals causing reproductive toxicity[J]. Asian Journal of Ecotoxicology, 2015, 10(1):31-39(in Chinese)
Guo L, Li Z Y, Gao P, et al. Ecological risk assessment of bisphenol A in surface waters of China based on both traditional and reproductive endpoints[J]. Chemosphere, 2015, 139:133-137 Liu N, Wang Y Y, Yang Q, et al. Probabilistic assessment of risks of diethylhexyl phthalate (DEHP) in surface waters of China on reproduction of fish[J]. Environmental Pollution, 2016, 213:482-488 Kamo M, Naito W. A novel approach to determining a population-level threshold in ecological risk assessment:A case study of zinc[J]. Human and Ecological Risk Assessment, 2008, 14(4):714-727 Rohr J R, Salice C J, Nisbet R M. The pros and cons of ecological risk assessment based on data from different levels of biological organization[J]. Critical Reviews in Toxicology, 2016, 46(9):756-784 Klimisch H J, Andreae M, Tillmann U. A systematic approach for evaluating the quality of experimental toxicological and ecotoxicological data[J]. Regulatory Toxicology and Pharmacology, 1997, 25(1):1-5 Duboudin C, Ciffroy P, Magaud H. Effects of data manipulation and statistical methods on species sensitivity distributions[J]. Environmental Toxicology and Chemistry, 2004, 23(2):489-499 Xu F L, Li Y L, Wang Y, et al. Key issues for the development and application of the species sensitivity distribution (SSD) model for ecological risk assessment[J]. Ecological Indicators, 2015, 54:227-237 Zhao J S, Chen B Y. Species sensitivity distribution for chlorpyrifos to aquatic organisms:Model choice and sample size[J]. Ecotoxicology and Environmental Safety, 2016, 125:161-169 Jin X W, Zha J M, Xu Y P, et al. Derivation of aquatic predicted no-effect concentration (PNEC) for 2,4-dichlorophenol:Comparing native species data with non-native species data[J]. Chemosphere, 2011, 84(10):1506-1511 Wang X N, Yan Z G, Liu Z T, et al. Comparison of species sensitivity distributions for species from China and the USA[J]. Environmental Science and Pollution Research, 2014, 21(1):168-176 Dyer S D, Versteeg D J, Belanger S E, et al. Interspecies correlation estimates predict protective environmental concentrations[J]. Environmental Science & Technology, 2006, 40(9):3102-3111 Wu F C, Mu Y S, Chang H, et al. Predicting water quality criteria for protecting aquatic life from physicochemical properties of metals or metalloids[J]. Environmental Science & Technology, 2012, 47(1):446-453 Jin X W, Wang Z J, Wang Y Y, et al. Do water quality criteria based on nonnative species provide appropriate protection for native species?[J]. Environmental Toxicology and Chemistry, 2015, 34(8):1793-1798 Wang B, Yu G, Huang J, et al. Tiered aquatic ecological risk assessment of organochlorine pesticides and their mixture in Jiangsu reach of Huaihe River, China[J]. Environmental Monitoring and Assessment, 2009, 157(1-4):29-42 Newman M C, Ownby D R, Mézin L C A, et al. Applying species-sensitivity distributions in ecological risk assessment:Assumptions of distribution type and sufficient numbers of species[J]. Environmental Toxicology and Chemistry, 2000, 19(2):508-515 Grist E P M, Leung K M Y, Wheeler J R, et al. Better Bootstrap estimation of hazardous concentration thresholds for aquatic assemblages[J]. Environmental Toxicology and Chemistry, 2002, 21(7):1515-1524 Killick R, Eckley I. Changepoint:An R package for changepoint analysis[J]. Journal of Statistical Software, 2014, 58(3):1-19 Wheeler J R, Grist E P M, Leung K M Y, et al. Species sensitivity distributions:Data and model choice[J]. Marine Pollution Bulletin, 2002, 45(1-12):192-202 United States Environmental Protection Agency. National Recommended Water Quality Criteria[R]. Washington DC:Office of Water, Office of Science and Technology, 2006 United States Environmental Protection Agency. National Recommended Water Quality Criteria[R]. Washington DC:Office of Water, Office of Science and Technology, 2009 Wong L C, Kwok K W H, Leung K M Y, et al. Relative sensitivity distribution of freshwater planktonic crustaceans to trace metals[J]. Human and Ecological Risk Assessment, 2009, 15(6):1335-1345 孔祥臻, 何伟, 秦宁, 等. 重金属对淡水生物生态风险的物种敏感度分布评估[J]. 中国环境科学, 2011, 31(9):1555-1562 Kong X Z, He W, Qin N, et al. Assessing acute ecological risks of heavy metals to freshwater organisms by species sensitivity distributions[J]. China Environmental Science, 2011, 31(9):1555-1562(in Chinese)
杜建国, 赵佳懿, 陈彬, 等. 应用物种敏感度分布评估重金属对海洋生物的生态风险[J]. 生态毒理学报, 2013, 8(4):561-570 Du J G, Zhao J Y, Chen B, et al. Assessing ecoloigical risks of heavy metals to marine organisms by species sensitivity distributions[J]. Asian Journal of Ecotoxicology, 2013, 8(4):561-570(in Chinese)
Levy J L, Stauber J L, Jolley D F. Sensitivity of marine microalgae to copper:The effect of biotic factors on copper adsorption and toxicity[J]. Science of the Total Environment, 2007, 387(1-3):141-154 Ankley G T, Bennett R S, Erickson R J, et al. Adverse outcome pathways:A conceptual framework to support ecotoxicology research and risk assessment[J]. Environmental Toxicology and Chemistry, 2010, 29(3):730-741 Wang Y, Na G S, Zong H M, et al. Applying adverse outcome pathways and species sensitivity-weighted distribution to predicted-no-effect concentration derivation and quantitative ecological risk assessment for bisphenol A and 4-nonylphenol in aquatic environments:A case study on Tianjin City, China[J]. Environmental Toxicology and Chemistry, 2018, 37(2):551-562 Maltby L, Blake N, Brock T C M, et al. Insecticide species sensitivity distributions:Importance of test species selection and relevance to aquatic ecosystems[J]. Environmental Toxicology and Chemistry, 2005, 24(2):379-388 Zhang L M, Wei C D, Zhang H, et al. Criteria for assessing the ecological risk of nonylphenol for aquatic life in Chinese surface fresh water[J]. Chemosphere, 2017, 184:569-574 -
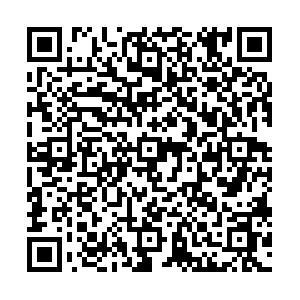
计量
- 文章访问数: 2274
- HTML全文浏览数: 2274
- PDF下载数: 115
- 施引文献: 0